Half Day Morning
Grand Ballroom ABCD
This tutorial is oriented toward administrators and developers who manage and use large-scale storage systems. An important goal of the tutorial is to give the audience the foundation for effectively comparing different storage system options, as well as a better understanding of the systems they already have.
Cluster-based parallel storage technologies are used to manage millions of files, thousands of concurrent jobs, and performance that scales from 10s to 100s of GB/sec. This tutorial will examine current state-of-the-art high-performance file systems and the underlying technologies employed to deliver scalable performance across a range of scientific and industrial applications.
The tutorial starts with a look at storage devices and SSDs in particular, which are growing in importance in all storage systems. Next we look at how a file system is put together, comparing and contrasting SAN file systems, scale-out NAS, object-based parallel file systems, and cloud-based storage systems.
- SSD technology
- Scaling the data path
- Scaling metadata
- Fault tolerance
- Manageability
- Cloud storage
Specific systems are discussed, including Ceph, Lustre, GPFS, PanFS, HDFS (Hadoop File System), BigTable, LevelDB, and Google's Colossus File System
Brent Welch, Google
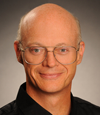
Brent Welch is a senior staff software engineer at Google, where he works on their public Cloud platform. He was Chief Technology Officer at Panasas and has also worked at Xerox-PARC and Sun Microsystems Laboratories. Brent has experience building software systems from the device driver level up through network servers, user applications, and graphical user interfaces. While getting his Ph.D. at the University of California, Berkeley, Brent designed and built the Sprite distributed file system. He is the creator of the TclHttpd web server, the exmh email user interface, and the author of Practical Programming in Tcl and Tk.
Irfan Ahmad, CachePhysics
Grand Ballroom EFGH
For a very long time, practical scaling of every level in the computing hierarchy has required innovation and improvement in caches. This is as true for CPUs as it is for storage and networked, distributed systems. As such, research into cache efficiency and efficacy improvements has been highly motivated and continues with strong improvements to this day. However, there are certain areas in cache algorithms optimization that have only recently experienced breakthroughs.
In this tutorial, we will start by reviewing the history of the caching algorithm research and practice in industry. Of particular interest to us are multi-tier memory hierarchies that are getting more complex and deep due to hardware innovations. These hierarchies and the workloads they generate motivate revisiting multi-tier algorithms. We will then describe cache utility curves and review recent literature that has made them easier to compute. Using this tool, we will excavate around caching policies and their trade-offs in different contexts. We will also spend some time thinking about optimality for caches.
- Overview and history of the caching algorithm research and practice in industry
- Introduction to new challenges posed by multi-tier memory hierarchies
- Review of cache utility curves and recent literature
- Experimenting with caching policies for production uses cases
- How to find the optimal cache
Ymir Vigfusson, Emory University

Ymir Vigfusson is Assistant Professor of Mathematics and Computer Science at Emory University since 2014, Adjunct Assistant Professor at the School of Computer Science at Reykjavik University since 2011, and a co-founder and Chief Science Officer of the offensive security company Syndis since 2013. Ymir completed his Ph.D. in Computer Science at Cornell University in 2010 where his dissertation on "Affinity in Distributed Systems" was nominated for the ACM Doctoral Dissertation Award.
His primary research interests are on distributed systems and caching, having worked on cache replacement in the IBM Websphere eXtreme Scale at IBM Research (2009–2011), and more recently as part of his NSF CAREER program on "Rethinking the Cache Abstraction." He has published at conferences that include ACM SOCC, USENIX ATC, VLDB, and EuroSys, as well as ACM TOCS. Ymir serves on the steering committee of LADIS (2010–2018), has been on program committees for ACM SOCC, ICDCS, EuroSys, and P2P. In addition to caching, Ymir also works on improving epidemiological surveillance and information security, funded by the Center for Disease Control and grants from the Icelandic Center for Research.
Irfan Ahmad, CachePhysics

Irfan Ahmad is the CEO and Cofounder of CachePhysics. Previously, he served as the CTO of CloudPhysics, pioneer in SaaS Virtualized IT Operations Management, which he cofounded in 2011. Irfan was at VMware for nine years, where he was R&D tech lead for the DRS team and co-inventor for flagship products including Storage DRS and Storage I/O Control. Before VMware, Irfan worked on the Crusoe software microprocessor at Transmeta.
Irfan is an inventor on more than 35 patents. He has published at ACM SOCC, FAST, USENIX ATC, and IEEE IISWC, including two Best Paper Awards. Irfan has chaired HotStorage, HotCloud and VMware’s R&D Innovation Conference. He serves on steering committees for HotStorage, HotCloud, and HotEdge. Irfan has served on program committees for USENIX ATC, FAST, MSST, HotCloud, and HotStorage, among others, and as a reviewer for the ACM Transactions on Storage.
Half Day Afternoon
Andy Rudoff, Intel
Grand Ballroom ABCD
Persistent Memory (“PM”) support is becoming ubiquitous in today’s operating systems and computing platforms. From Windows to Linux to open source, and from NVDIMM, PCI Express, storage-attached and network-attached interconnect access, it is available broadly across the industry. Its byte-addressability and ultra-low latency, combined with its durability, promise a revolution in storage and applications as they evolve to take advantage of these new platform capabilities.
Our tutorial explores the concepts and today’s programming methodologies for PM, including the SNIA NonVolatile Memory Programming Model architecture, open source and native APIs, operating system support for PM such as direct access filesystems, and via language and compiler approaches as well. The software PM landscape is already rich, and growing.
Additionally, the tutorial will explore the considerations when PM access is extended across fabrics such as networks, I/O interconnects, and other non-local access. While the programming paradigms remain common, the implications on latency, protocols, and especially error recovery are critically important to both performance and correctness. Understanding these requirements are of interest to both the system and application developer or designer.
Specific programming examples, fully functional on today’s systems, will be shown and analyzed. Concepts for moving new applications and storage paradigms to PM will be motivated and explored. Application developers, system software developers, and network system designers will all benefit. Anyone interested in an in-depth introduction to PM in emerging software and hardware systems can also expect an illuminating and thought-provoking experience.
- Persistent memory
- Persistent memory technologies
- Remote persistent memory
- Programming interfaces
- Operating systems
- Open source libraries
- RDMA
Tom Talpey, Microsoft
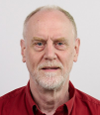
Tom Talpey is an Architect in the Networking team at Microsoft Corporation in the Windows Devices Group. His current areas of focus include RDMA networking, remote filesharing, and persistent memory. He is especially active in bringing all three together into a new ultra-low-latency remote storage solution, merging the groundbreaking advancements in network and storage-class memory latency. He has over 30 years of industry experience in operating systems, network stacks, network filesystems, RDMA and storage, and is a longtime presenter and instructor at diverse industry events.
Andy Rudoff, Intel
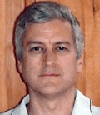
Andy Rudoff is a Principal Engineer at Intel Corporation, focusing on Non-Volatile Memory programming. He is a contributor to the SNIA NVM Programming Technical Work Group. His more than 30 years industry experience includes design and development work in operating systems, file systems, networking, and fault management at companies large and small, including Sun Microsystems and VMware. Andy has taught various Operating Systems classes over the years and is a co-author of the popular UNIX Network Programming text book.
Grand Ballroom EFGH
Enterprises today have a plethora of information that needs to be harnessed for business insights. Over the years, Enterprises have made investments in a variety of storage solutions, relational databases, warehouses, NoSQL stores, Big Data analytics platforms, Data Lakes, Cloud Stores, etc. As we enter the era of Machine Learning (ML), it is important to understand how to bring these silos together to discover, build, and deploy ML models in production.
This tutorial covers the technical concepts and architectural models required to operationalize and architect your Enterprise Data Fabric for ML initiatives. The tutorial is divided into the following sections:
- A Data Engineering perspective on the end-to-end ML workflow in-production
- Taxonomy of requirements & landscape of available building blocks for the Data Fabric
- Putting it together: Defining the Data Fabric architecture with reference examples
The tutorial assumes a basic knowledge of popular Big Data and Analytics solutions. We assume no ML background—our focus will be on operational concepts rather than the internal mathematical formulations of ML algorithms. The tutorial is designed for Storage architects, Data Engineers, and Engineering Managers interested in learning designing of Data Fabrics.
- Different architectures for Data Stores (Relational, MPP, NoSQL, Event Stores, In-memory grids, etc.)
- Different Analytic programming models and Frameworks (Batch, Interactive, Stream)
- Example Cloud computing platforms for Data Management
- Workflow for Machine Learning models in production
- Blue-print of a Data Fabric
- Examples reference architectures of Data Fabric deployments
Sandeep Uttamchandani, Intuit

Sandeep Uttamchandani is a Distinguished Engineer at Intuit, focussing on platforms for storage, databases, analytics, and machine learning. Prior to Intuit, Sandeep was co-founder and CEO of a machine learning startup focussed on finding security vulnerabilities in Cloud Native deployment stacks. Sandeep has nearly two decades of experience in storage and data platforms, and has held various technical leadership roles at VMware and IBM. Over his career, Sandeep has contributed to multiple enterprise products, and holds 35+ issued patents, 20+ conference and journal publications, and regularly blogs on All-things-Enterprise-Data. He has a Ph.D. from University of Illinois at Urbana-Champaign.
Continuing Education Units (CEUs)
USENIX provides Continuing Education Units for a small additional administrative fee. The CEU is a nationally recognized standard unit of measure for continuing education and training and is used by thousands of organizations.
Two half-day tutorials qualify for 0.6 CEUs. You can request CEU credit by completing the CEU section on the registration form. USENIX provides a certificate for each attendee taking a tutorial for CEU credit. CEUs are not the same as college credits. Consult your employer or school to determine their applicability.
Training Materials on USB Drives
Training materials will be provided to you on an 8GB USB drive. If you'd like to access them during your class, remember to bring a laptop.